Clear as Mud: An Attempt to Demystify HUD’s CDBG Funding Algorithm
As spaces are becoming increasingly quantified and modeled, data’s swift trajectory to the highest levels of decision-making speak to its formidable position in urban processes. In the United States, the largest algorithmic decision-making processes take place in multi-part and massive scales, and such processes have to be studied as a whole. In particular, federal allotment processes entail the use of national demographic datasets and the application of statistical models to calculate community needs across the nation. This indisputably presents ample room for oversimplification, modelling errors, and disconnect from lived experiences. These concerns surrounding the uncertainty of the data apparatus as a whole have substantiated a call for reproducibility. In this study, HUD’s Community Development Block Grant (CDBG) Entitlement program was evaluated in terms of its reproducibility, data accessibility, transparency, and democratization. Through a close reading of public records and designing a GIS-based model to recreate HUD’s 2021 CDBG Entitlement allocations based on available data, this thesis identifies the ways in which the program’s allocations are irreproducible: its methodology and data lack transparency to the extent that is not possible for independent or third-party researchers to conduct crucial analyses on the algorithm’s inputs variables, sampling error, or effectiveness of the funds.
This study also argues that the CDBG Entitlement program’s opaque procedures limit democratic discourse and perpetuate the co-institutions of data and federal power.
View the presentation deck:



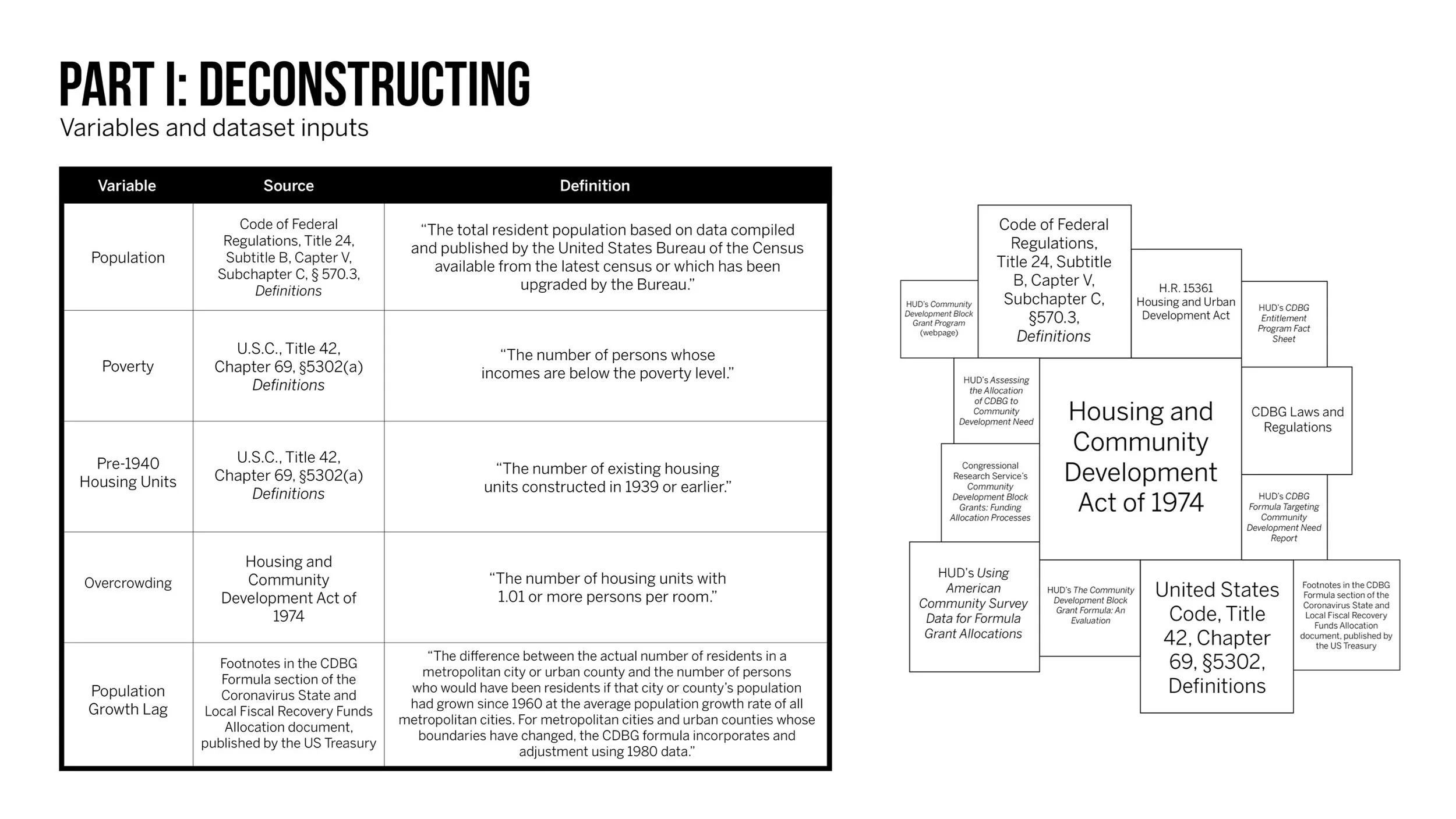

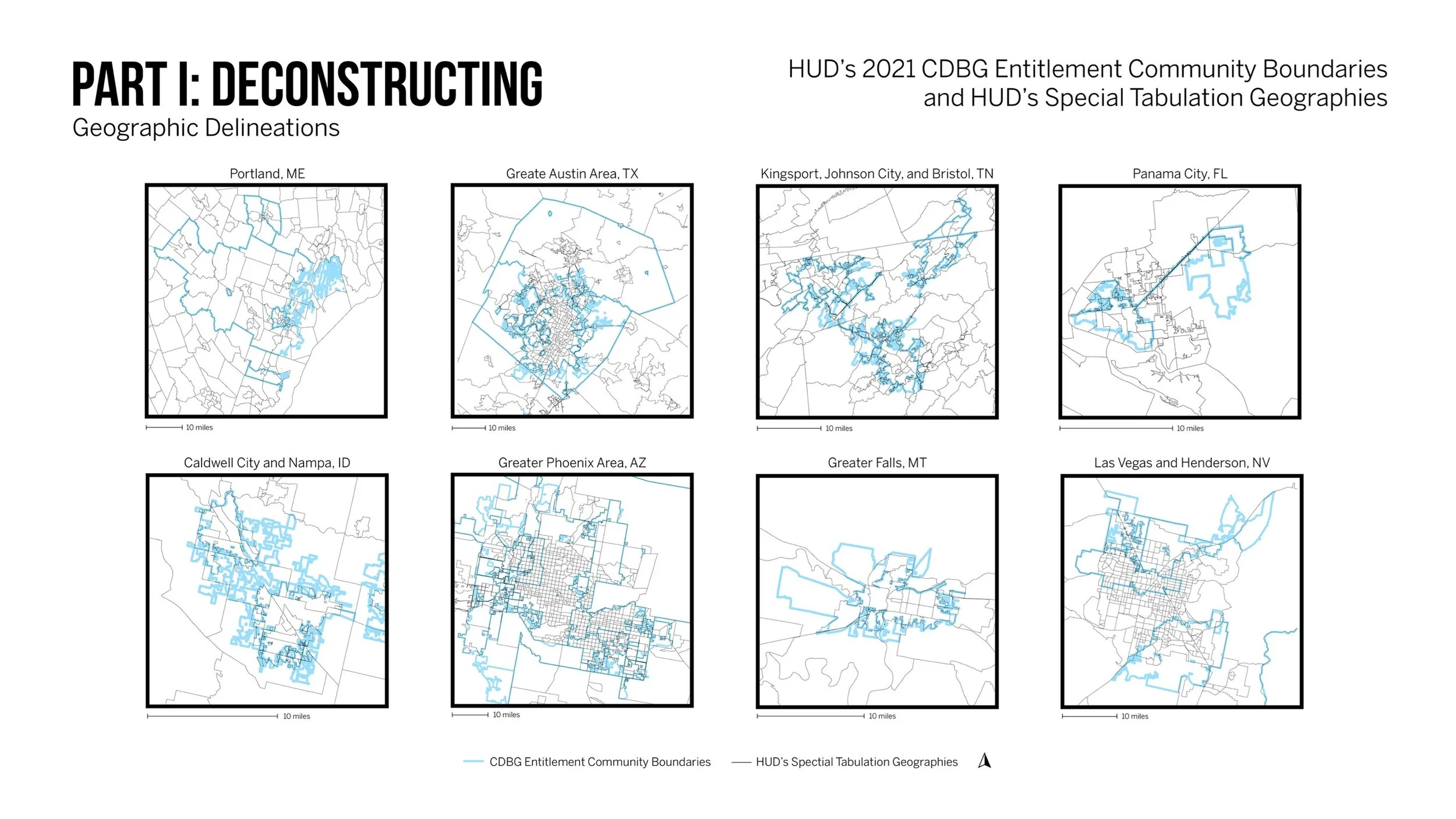

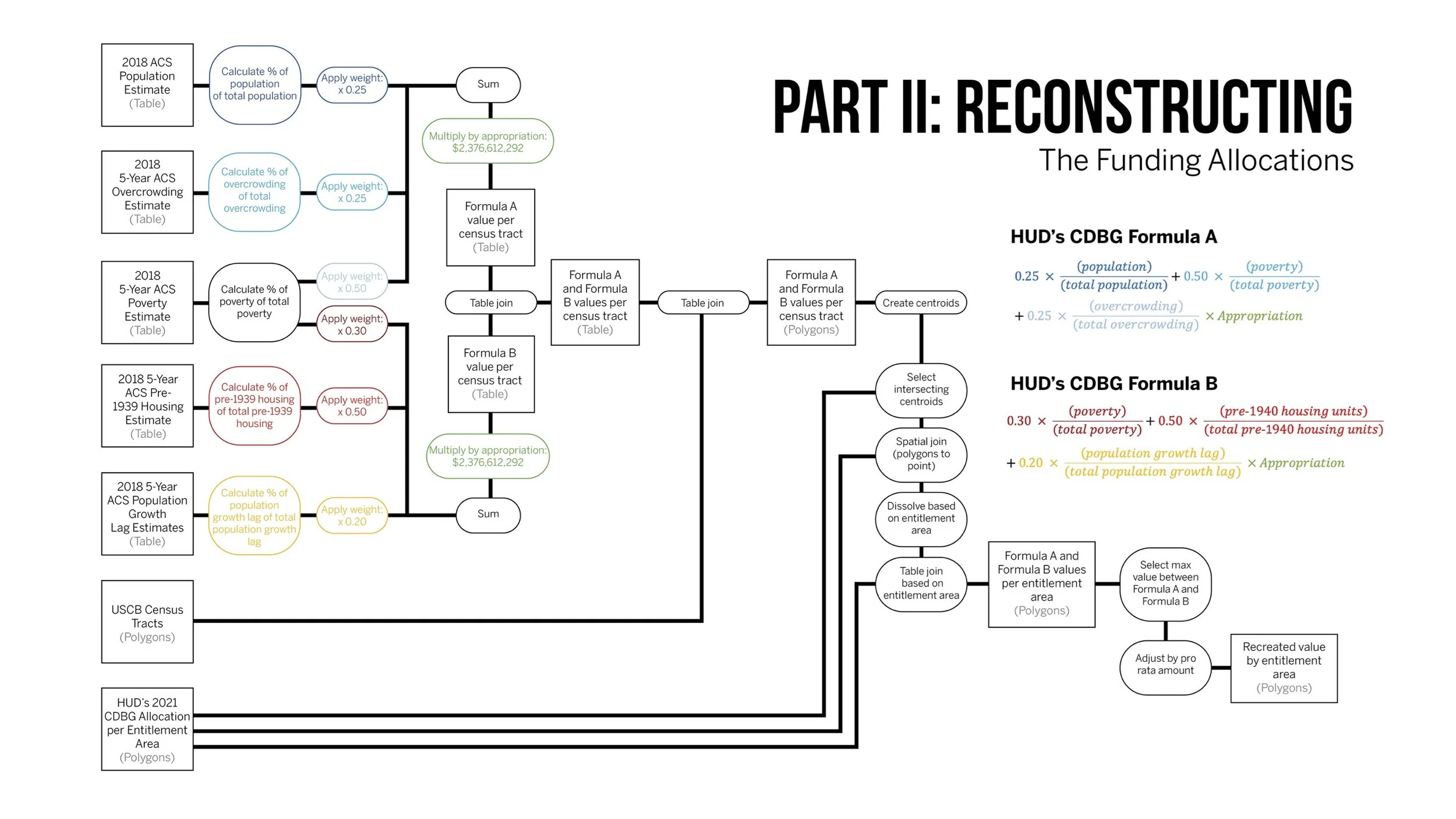
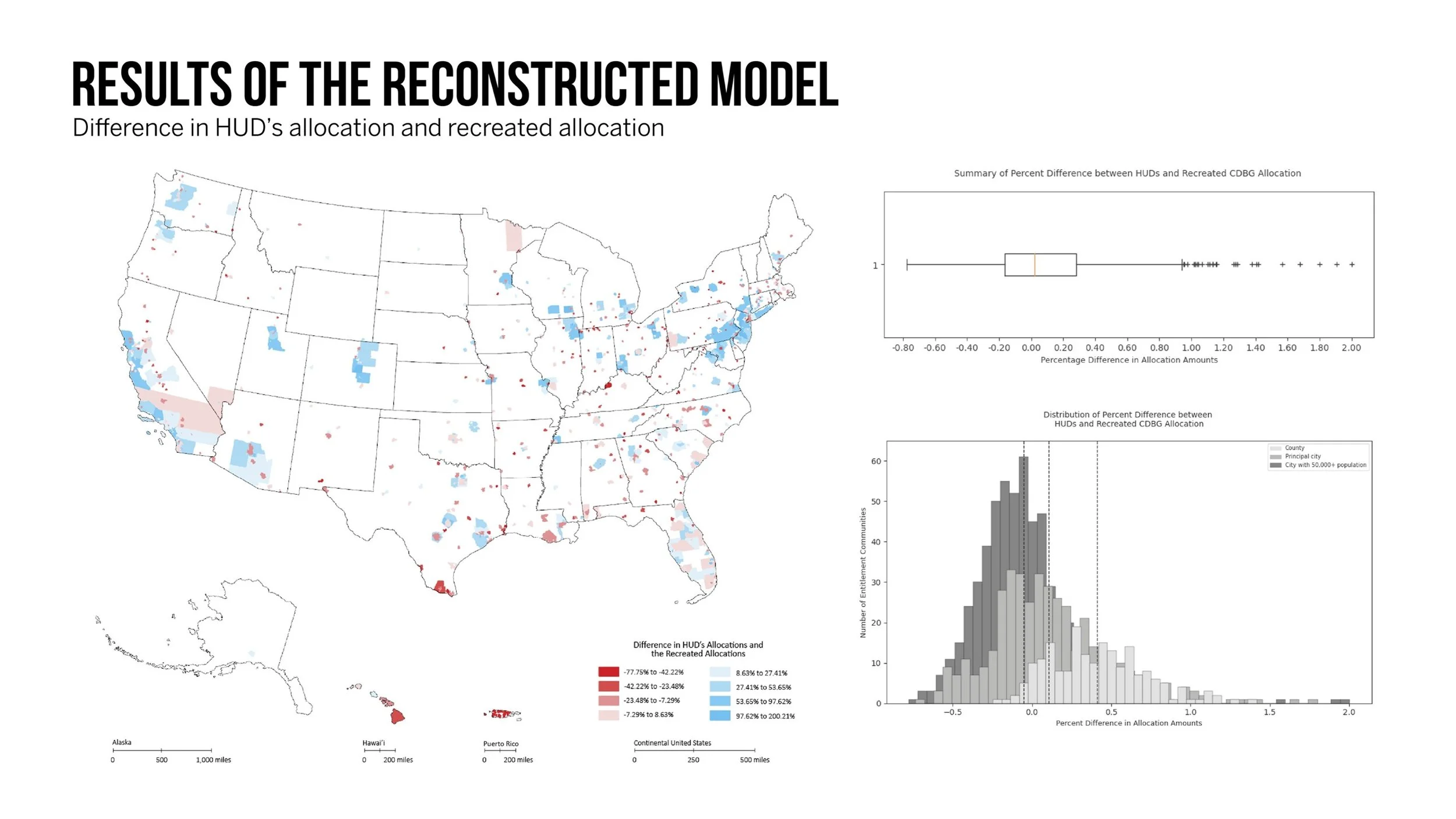


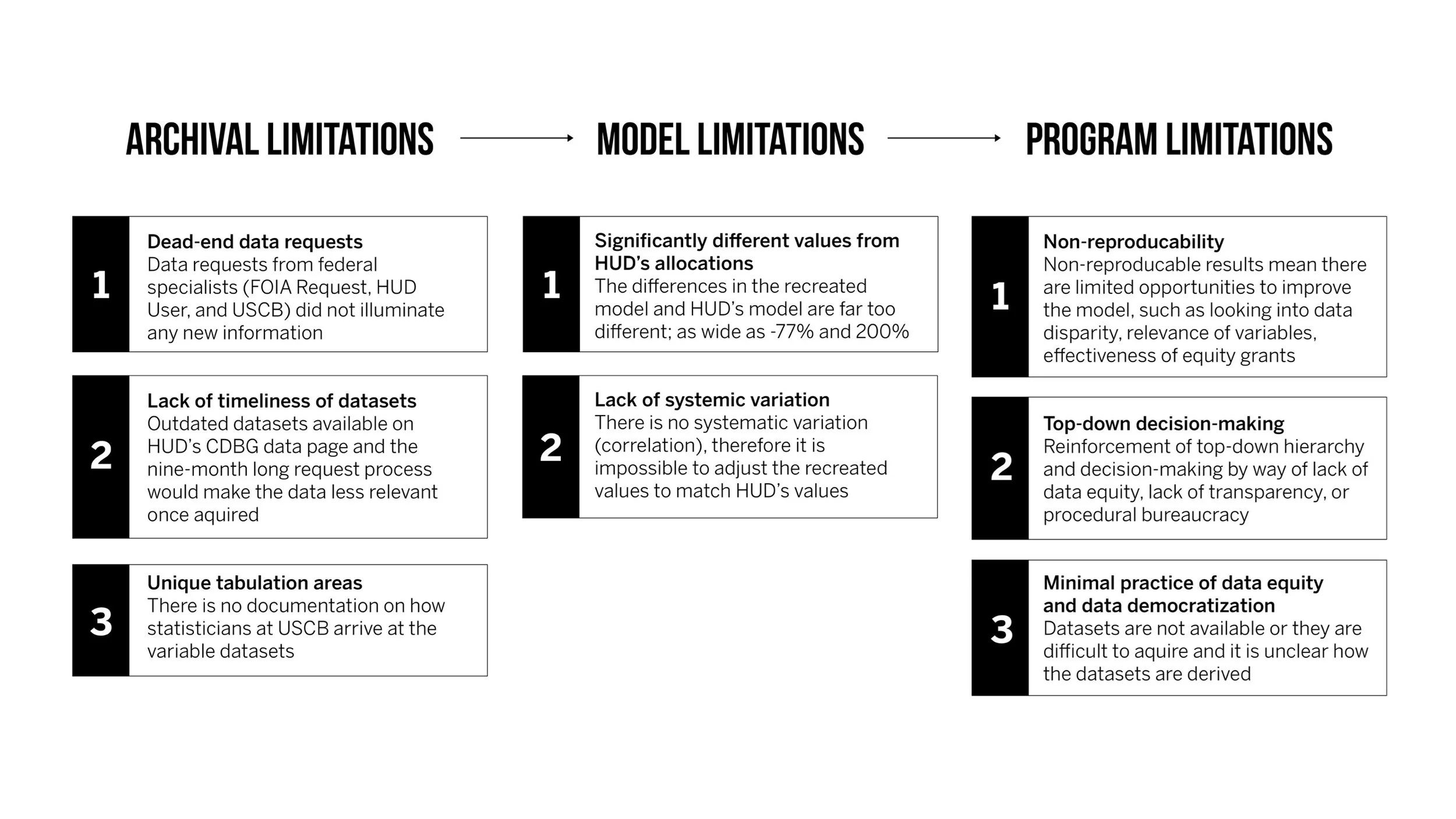

